Best of Generative AI Research this week
We have handpicked some of the best Generative AI research work published this week so that you don't have to spend ages surfing and sifting through pages of articles, drowning in the ever-growing AI literature.
HyperHuman: Hyper-Realistic Human Generation with Latent Structural Diffusion
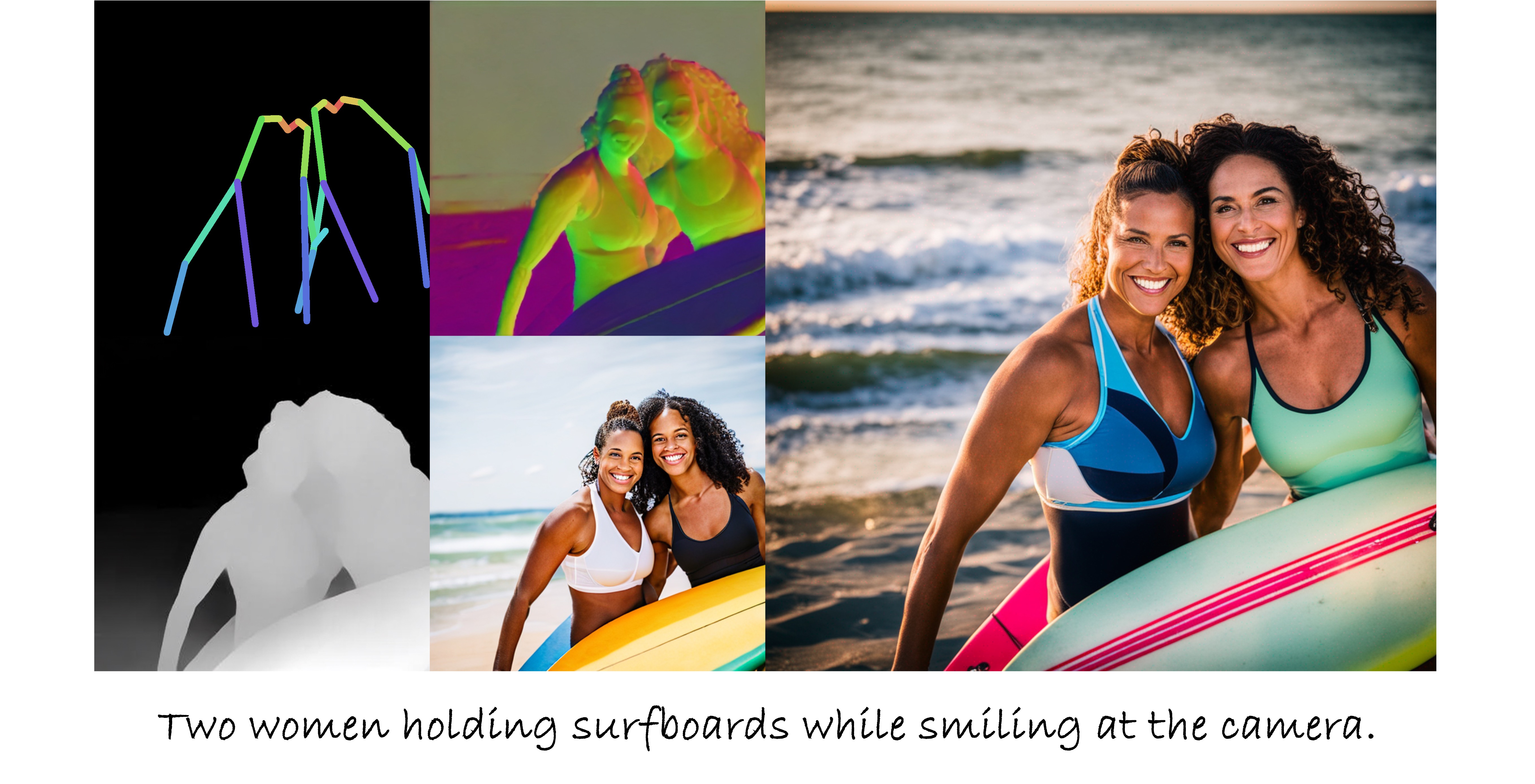
What if I told you the above two humans don't exist for real?
This is what exactly has been achieved by the HyperHuman paper from Snap. HyperHuman, generates in-the-wild human images of high realism and diverse layouts. Existing models like Stable Diffusion and DALL·E 2 tend to generate human images with incoherent parts or unnatural poses. Capturing correlations between the explicit appearance and latent structure in one model is essential to generating coherent and natural human images. To achieve this:
- They first created HumanVerse, a dataset that consists of 340M images with comprehensive annotations like human pose, depth, and surface-normal.
- They propose a Latent Structural Diffusion Model that simultaneously denoises the depth and surface-normal along with the synthesized RGB image.
- Then a Structure-Guided Refiner composes the predicted conditions for more detailed generation of higher resolution.
Have a look at the paper yourself here
LAMP: Learn A Motion Pattern for Few-Shot-Based Video Generation
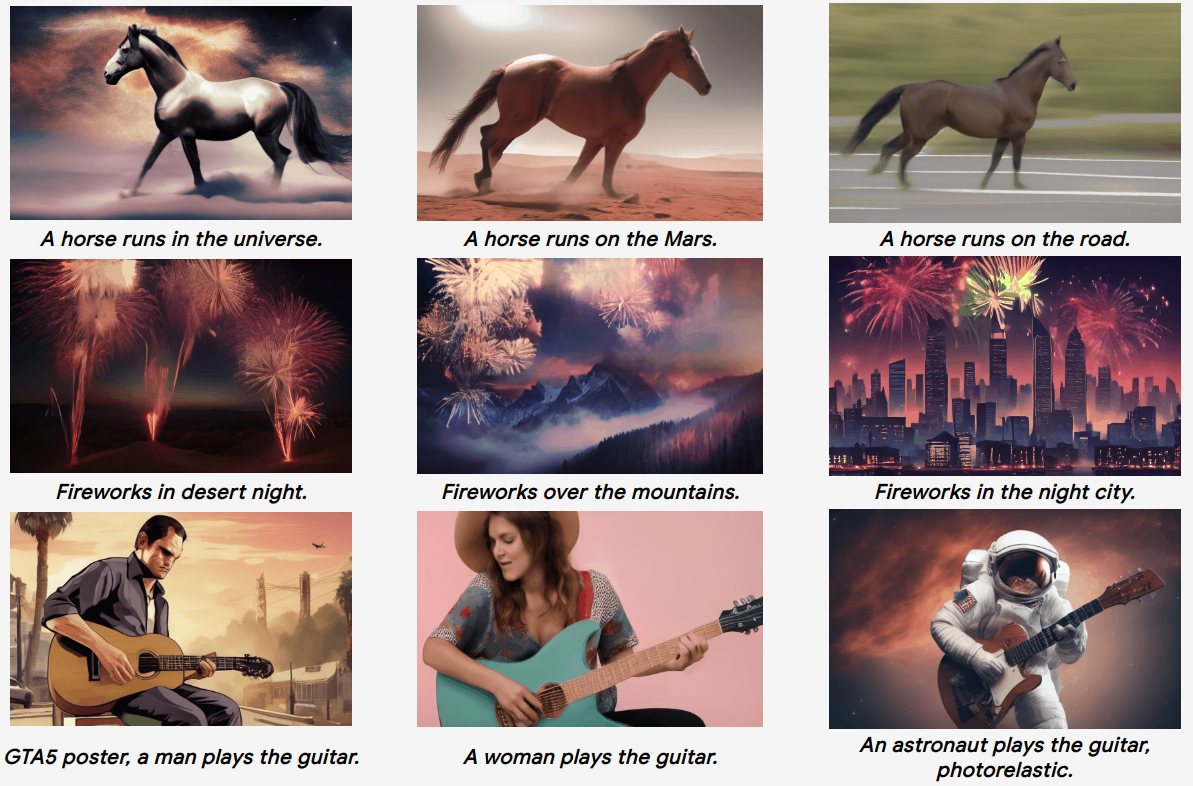
While HyperHuman is for images, LAMP is the newest Video generation model in town. How does it work?
- LAMP learns a motion pattern from a small video set and generates videos with the learned motion, which achieves the trade-off between training burden and generation freedom.
- LAMP transfers text-to-video generation to the first-frame generation and subsequent-frame prediction, i.e., decoupling a video's contents and motions.
- During the training stage, noise is added and loss is computed on all but the first frame. Moreover, only the parameters of newly added layers and the query linear layers of self-attention blocks are updated when tuning.
- During the inference stage, a T2I model is used to generate the first frame. The tuned model only works on denoising the latent features of subsequent frames with the guidance of user prompts.
To fully read the paper head here
Progressive3D: Progressively Local Editing for Text-to-3D Content Creation with Complex Semantic Prompts
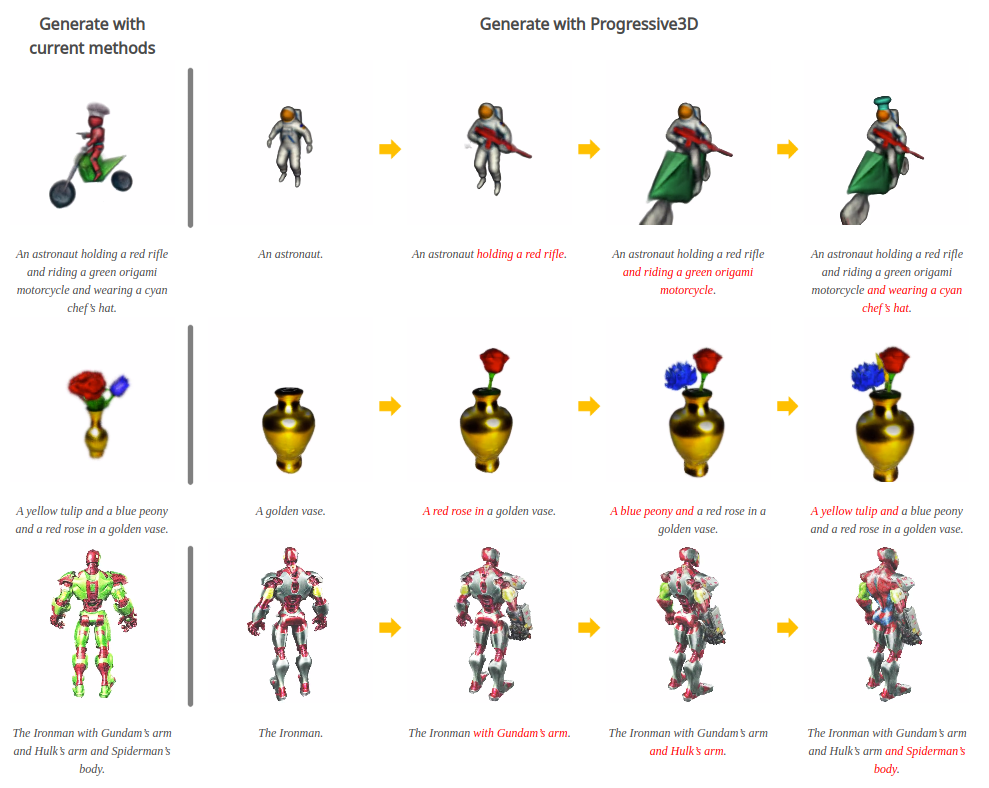
Let's shift our attention (not the one in transformers) to 3D. Though 3D content generation has come a long way, current methods struggle to generate correct 3D content for a complex prompt in semantics, i.e., a prompt describing multiple interacted objects binding with different attributes. To address this problem, Progressive3D proposes:
- To decompose the entire generation into a series of locally progressive editing steps to create precise 3D content for complex prompts
- constrain the content change to occur only in regions determined by user-defined region prompts
- use a semantic component suppression technique to encourage the optimization process to focus more on the semantic differences between prompts
To fully understand the paper, please read it here
4K4D: Real-Time 4D View Synthesis at 4K Resolution
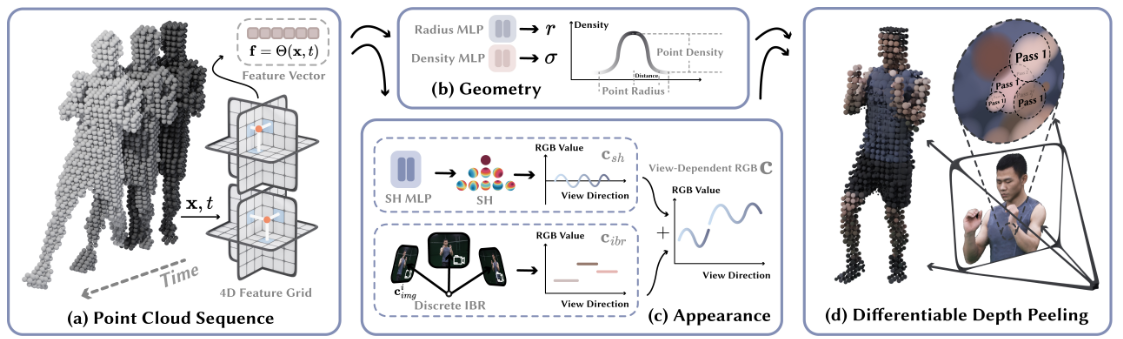
As if generating videos and 3D data is not mind-blowing, we have 4D generation now. That too at 4K resolution! Yes its 4K4D as the name says! And what not, its real-time! Here is how it goes:
- 4K4D is a 4D point cloud representation that supports hardware rasterization and enables unprecedented rendering speed. Its a novel hybrid appearance model that significantly boosts the rendering quality while preserving efficiency
- First a space-carving algorithm, we extract the initial cloud sequence x,t of the target scene. A 4D feature grid is predefined to assign a feature vector to each point, which is then fed into MLPs for the scene geometry and appearance.
- Next, the geometry model is based on the point location, radius, and density, which forms a semi-transparent point cloud. % and spherical harmonics coefficients
- Next, The appearance model consists of a piece-wise constant IBR term
- Finally, the proposed representation is learned from multi-view RGB videos through the differentiable depth peeling algorithm.
Checkout the paper here
Hope that gave a nice snapshot of the week. Have a good weekend and I will see you in my next post and video.
Talking of video, please subscribe to our YouTube channel if you haven't already done. Also checkout our latest video on Mistral AI's 7 billion parameters model: